Colliders play a crucial role in scientific research, particularly in physics and statistics, but they are often misunderstood. So, what exactly is a collider? This ultimate guide will explore everything you need to know about colliders and their impact on data analysis and scientific experiments.
What Is a Collider? Understanding the Basics
A collider is a node where multiple causal pathways converge, significantly influencing the correlation between variables. A collider occurs in a scenario where two or more causal influences converge on a single point, known as a node.
For example, consider a situation where both your genetic disposition and your diet contribute to your health; here, health acts as a collider between these two variables. This setup can lead to what is known as a spurious correlation if not properly managed during analysis.
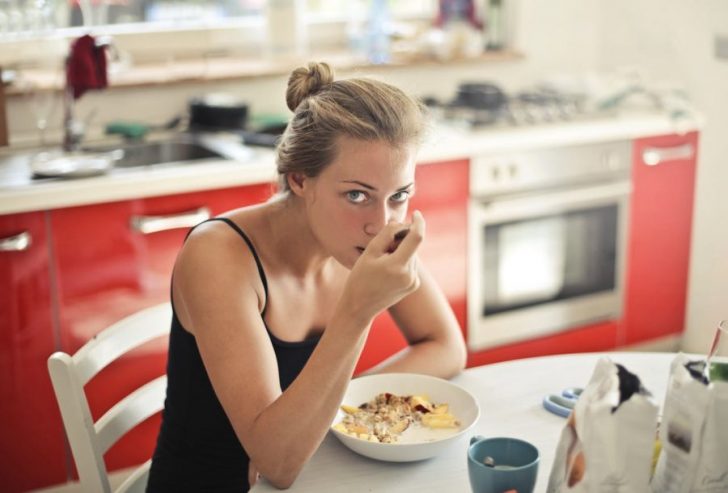
Andrea Piacquadio | Pexels | Consider a situation where both your genetic disposition and your diet contribute to your health; here, health acts as a collider between these two variables.
How Colliders Affect Research
The primary challenge with colliders is that they can obscure the true relationship between variables. When variables converge at a collider, it can create or mask associations that do not exist in other circumstances. This phenomenon complicates the task of distinguishing genuine causal connections from mere correlations.
Take, for instance, the classic case study in which researchers observed that taking certain medications correlated with specific health outcomes. At first glance, the medication directly influences health. However, if a third variable, like age, influences both medication intake and health outcomes without proper controls in place, the study could lead to incorrect conclusions.
The Role of Statistical Control in Managing Colliders
In statistical analysis, controlling for colliders is essential. By adjusting for the effects of a collider, researchers can block non-causal paths that would otherwise suggest a correlation. This adjustment is critical in experiments where researchers aim to isolate the effect of specific variables.
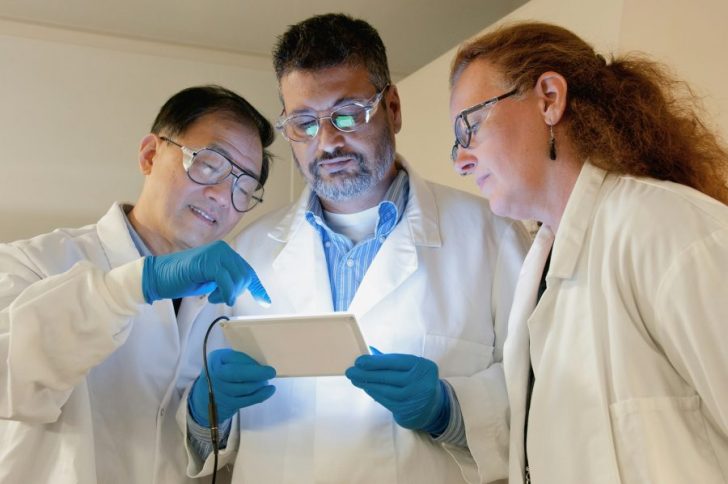
National Cancer Institute | Unsplash | By adjusting for the effects of a collider, researchers can block non-causal paths that would otherwise suggest a correlation.
For example, in randomized control trials, the assignment of treatment is randomized to ensure that the observed effects can be attributed solely to the treatment, thereby eliminating the influence of colliders.
Practical Examples of Collider Effects
Consider a research scenario involving the impact of educational tools on student performance, with motivation as a potential collider. If both the quality of educational tools and the intrinsic motivation of students affect performance, failing to account for motivation could lead to misleading interpretations of the data. Researchers might conclude that the tools have a significant impact when, in fact, much of the effect could be attributed to student motivation.
In another example, imagine researchers studying the relationship between diet and exercise on weight loss, with metabolism acting as a collider. Without accounting for individual metabolic differences, the study might incorrectly attribute weight loss results solely to diet or exercise.
Collider Identification and Its Importance
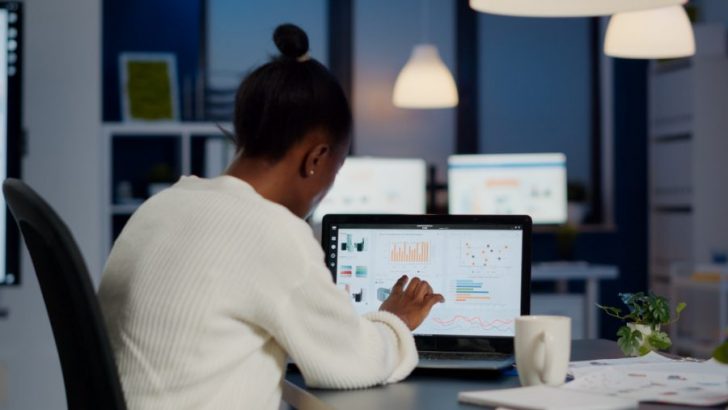
DC Studio | Freepik | Tools like statistical software are invaluable in helping researchers visualize and control for colliders, ensuring that their analyses yield accurate and reliable conclusions.
Identifying colliders is not merely an academic exercise; it has practical implications in fields ranging from epidemiology to economics. Tools like causal diagrams and statistical software are invaluable in helping researchers visualize and control for colliders, ensuring that their analyses yield accurate and reliable conclusions.
Understanding and controlling for colliders is not just a technical detail in research methodology; it is fundamental to achieving valid and actionable insights. Colliders can dramatically alter the interpretation of data, and recognizing their role enables researchers to conduct more precise and meaningful studies.
Whether you are a seasoned scientist or a student just beginning to explore the complexities of research, grasping the concept of colliders is a step towards more robust and credible scientific inquiry.